The Future of Machine Learning: Expertise, Experience, and Building Trust
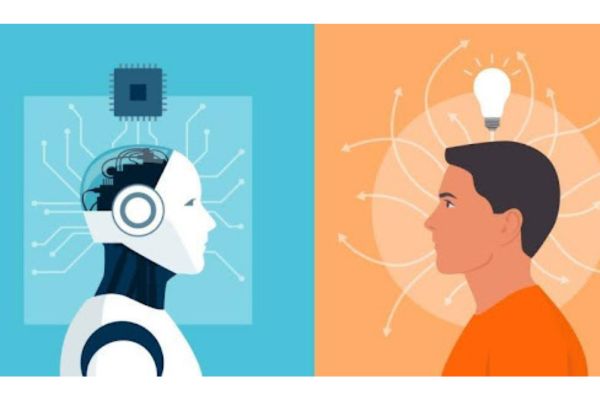
From recommending movies to predicting the weather, Machine Learning (ML) is changing our world. But what’s next for it? In what ways will ML develop, too how can we make certain that it’s trustworthy in addition reliable? This is where the ideas of Expertise, Experience, Authoritativeness, in addition, Trustworthiness (EEAT) come into play.
Think of ML as a student:
Expertise: Think of a student who has a deep understanding of a single subject. In the future Machine Learning Development will be even more specialized than now, excelling in certain areas. For example, an ML system might become an expert in diagnosing diseases or in predicting traffic flow for some cities.
Experience: Like a student who acquires experience by doing, future ML will “gain experience” by processing huge amounts of data. Consequently, it will be able to make more accurate predictions and find patterns that people overlook. Imagine an ML system examining millions of medical scans, and gaining for a doctor the equivalent of years’ experience.
Building Trustworthy AI Partners:
Now let’s talk about trust. Imagine a student who couldn’t be trusted with a decisive project. This is how ML can build trust:
Authoritativeness:
We trust information from reliable sources. Future ML systems will be able to cite their sources and explain their reasoning. Imagine an ML system recommending a movie not only says “watch this” but goes into why it suggested it after analyzing reviews and taking into account users’ preferences.
Trustworthiness:
Just as we trust a student to be honest, we need trustworthy ML. We will see advances in the detection of bias and in explainability. Explainable AI means we can know how an ML system concluded, adding confidence to its decisions. This could involve the ML itself pointing out potential biases in the data it used to reach that conclusion.
The Future is a Collaboration:
ML’s future lies not in replacing humans, but in partnership. Think of a student being mentored by a seasoned professional. Specialists in both fields will continue to supervise the development of ML, ensuring it remains in alignment with human values and that it solves real-world problems. For example, doctors might work with systems based on ML to develop better treatment plans.
Find here : Top 5 Best Language Learning Apps
EEAT in Action:
Here are some examples of how EEAT can be applied in the future:
Personalized Learning:
An ML system with expertise in education could tailor learning experiences for each student, providing a reliable tutor like the ones of the old. Experienced ones at that.
Cybersecurity:
Highly specialized ML systems could process tremendous volumes of data to identify and prevent cyber threats, serving as your data guard which is both authoritative and experienced.
The Road Ahead:
By promoting an understanding of Expertise, Experience, Authoritativeness, and Trustworthiness (EEAT), we can turn future ML solutions into friendly collaborators rather than mere tools. This collaborative future between people and machines is brimming with the potential to solve complex problems and create a better world.
The EEAT Imperative: Ensuring Responsible and Trustworthy Machine Learning Development Solutions in a Complex World
The last section discussed how Expertise, Experience, Authoritativeness, and Trustworthiness (EEAT) will affect the future of Machine Learning (ML). Now let’s look into what lies ahead.
Challenges and Considerations
Data Biases: ML is based on feeding data into systems, and biased data can lead to biased results. Ensuring fairness and inclusiveness in future solutions will thus require robust ways of spotting and correcting biases. Suppose the data being used to train an ML system for loan approvals contains a bias due to zip code. We must ensure that the data used in training the system reflects the true population of people.
Explainability and Transparency
As ML becomes more complex, understanding how it reaches conclusions is crucial. Explainable AI techniques will be essential for building trust and ensuring human oversight. Security and privacy
Just imagine an ML system used in self-driving cars. We need to understand why it makes specific decisions on the road for passenger security, an entirely complex issue.
Robust security measures will be needed to protect sensitive information and prevent misuse, both on the part of storeowners and seekers after goods who try to make shopping easier by scanning their faces instead of keying into a card-type device. Allowing data ownership and usage guidelines to be set out ensures user privacy is respected by product or service maintainers. So, imagine an ML system analyzing medical records. Account security protocols are in place to ensure the privacy of patients through the use of data totalization techniques for individual items of personal information.
Opportunities for Collaboration
Human-in-the-Loop Systems: The future lies in cooperation humans setting goals and guiding ML development on everything from data analysis to complex analyses, but ML is heavily involved in that work. Imagine doctors working together with ML systems to diagnose cancer, the doctor making the final judgment on what treatment to give based on an analysis by ML and his own experience.
Continuous learning and improvement are a continuous process. Human experts can play a crucial role in identifying areas for improvement and ensuring that the systems can adapt to changing environments.
Just imagine an ML system used for weather forecasting. As new weather patterns emerge, meteorologists guide the system in its adaptation efforts so that over time accuracy of predictions increases.
The Democratization of AI: The benefits of ML should not be confined to large enterprises. User-friendly tool development and open-source platforms can extend the outreach of artificial intelligence to smaller organizations or even individuals. This stimulates creativity and broadens EEAT into myriad potential applications. Let’s say you develop an ML system to monitor environmental changes in a local community that now helps those living there benefit from its insights and know-how. In practice, you no longer need to meet out of a glut for help under pressure generally giving resourceful and unconventional internet startups such as those used
The Benefits of Responsible ML:
If we make sure that EEAT takes priority, then the full potential of ML for doing good will be unlocked. Here are some of the fundamental advantages:
Better Decision-Making:
Reliable and trustworthy ML can provide valuable insights for decision-making in different areas. Imagine applying ML to plan traffic flows in a city creating time-related efficiencies of several minutes for each trip
Enhanced Efficiency and Increased Productivity:
The automation of routine tasks with ML leaves human resources free for more important things. For example, an ML system is used to deal with basic inquiries from customers who are tired of the wait, thus allowing the actual service personnel to concentrate on complicated problems.
Scientific Discovery and Innovation:
ML can identify patterns and connections within large pieces of data that humans might overlook, thus accelerating scientific breakthroughs. For example, ML could also be used to analyze genetic data developing new treatments for diseases with this potential
The Road to a Brighter Future
The future of machine learning development is headed in a positive direction, but communities need to build programs that are not only powerful but also ethical and trustworthy. By concentrating on Expertise, Experience, Authority, and Trustworthiness, we can guarantee that ML becomes a power for good – providing human society with a means to solve the most pressing questions that currently confront it.
In the future, as we have seen, the direction of machine learning development solutions will depend upon the application of EEAT principles. But underpinning this, essential to any picture that takes human intelligence together with Artificial Intelligence is blurring boundaries between experts and non-experts again So how do you envisage human experts interacting with machine learning solutions software? Try jotting down some innovative suggestions.
Why Humans Remain Essential:
Ethical considerations and guiding principles: Machine learning development is best utilized for data analysis only, it simply cannot make any decisions involving the moral compass and ethical judgment vested in human intelligence. Experts will continue to shape the guiding principles of ML development so that it aligns with human values without generating unexpected results. Take the case of the ML system used for facial recognition by the police. Experts would be vital in setting ethical guidelines to prevent any racial bias or violation of privacy.
Context and domain knowledge:
ML thrives on data yet live data lacks the nuances of human understanding. In the same way experts contribute specific knowledge in their fields of expertise and context, they also interpret data for the benefit of systems designing ML solutions However, ML solutions must solve real-world problems effectively. Imagine applying ML to predict crop yields. Agricultural experts can help the system understand that there are factors other than weather patterns that need to be considered, such as soil quality and pestilence.
Creativity and Innovation:
Human ingenuity uncovers the limit of ML. Experts will keep creating innovative applications, proposing open-ended experiments, and raising questions that reveal ML’s full potential. Imagine using ML to analyze historical texts. Historians moreover, when brought into contact with ML professionals, can develop a system that not only lists facts but also brings to light hitherto undetected connections among events.
Human-Centered Design for Trustworthy ML:
Interpretable User Interfaces:
Good communication is key to trust. Experts will be able to design the interface of a machine learning development solutions system so that it shows the reasoning behind its decision. Imagine an ML system used for approving loans. The system can show the user just what factors went into making this decision, offering transparency and trust.
Active Learning and Human-in-the-Loop Systems:
A student always learns from a teacher. Similarly, active learning future ML systems will benefit from human feedback shout and guide learning. This can mean constantly improving the training data, or it can mean human audit discovers another possible source of bias.
Focus on Human-Machine Collaboration:
The upcoming is not about replacing humans with machines, nonetheless rather building a partnership between the two. This way, by using each other’s strengths we can attain a great agreement. Imagine an ML-powered robotic assistant working alongside surgeons during complex surgery, adding together human skill and machine precision; the result speaks for itself.
Conclusion: A Symbiotic Future
The future of machine learning solutions lies in the relationship between humans and machines. To realize this ideal future, we should strive to follow EEAT principles and always encourage greater teamwork. In the end, we will all benefit as long as AI is developed humanely and in an atmosphere of mutual collaboration − benefiting from human guidance as well as input. This partnership between human beings and machines holds the key to a future where both work together for a better world.
FAQs about the Future of Machine Learning
What exactly is EEAT and how does it relate to Machine Learning?
EEAT stands for Expertise, Authoritativeness, and Trustworthiness. In the future of Machine learning solutions (ML), these key principles will be indispensable for ensuring that solutions made by ML systems are reliable, unbiased, and contribute to positive change.
What new specialties will ML expertise develop in the future?
Data are now processed in a scalable manner to enable future MLX systems to learn from their environment. This enables machines to identify patterns, take advantage of experience, and make predictions far more accurately than ever before. A future example is an ML program that will learn from millions of medical scans, the equivalent of years’ worth of a doctor’s experience hitting it all in one go.
Why is explainability important for trustworthy ML?
For users to trust ML, they need to understand how it reaches conclusions. Explainable AI techniques will allow us to understand the reasons behind an ML system’s decisions and therefore gain trust.
How can bias be mitigated in future ML?
Mitigating bias is essential if ML is to be fair and all-embracing in the future. Future solutions will involve robust mechanisms for detecting bias and possibly correcting it which are built into all data used to train. Think of a program that will ensure an ML system used for loan approvals doesn’t discriminate on grounds irrelevant to the applicant.
How will security and privacy be addressed in future ML?
As ML interacts with more and more data, there will be a need for increasingly robust security measures to protect sensitive information. Clear lines of ownership and use of data will also have to be drawn to prevent user privacy from being violated.
How can humans and machines collaborate effectively in the future?
The future lies in human-in-the-loop systems, where humans set goals and guide ML development, while ML does heavy data analysis. This prioritizes human values and ethical considerations.
How will continuous learning be implemented in future ML?
EEAT is a long-term process. ML systems need to be regularly monitored and experience acquired, as they improve. Human experts are indispensable for identifying areas of improvement and adapting systems to fill changes in their environment.
On the question of the future of ML, why not bring AI within reach of democracy?
If ML tools and platforms become more accessible, Pollyanna not tied to legacy source code can at least get their voices heard. This supports creative capabilities and broadens the consequences of EEAT principles. For example, perhaps in A community ML is used to monitor local environmental changes.
What are the main benefits of responsible ML regulated by Deathwatch responsible ML comes with enhanced decision-making across all fields, efficiencies, and productivity through automation, and is, a harbinger of faster scientific breakthroughs than Now that Google Earth has provided data at the touch of a button.